Japanese
English
- 有料閲覧
- Abstract 文献概要
- 1ページ目 Look Inside
- 参考文献 Reference
レディオミクスは2012年にLambinらによって提案され1),10年あまりの間に様々な疾患の予後などの予測に応用されてきた。対象疾患は多岐にわたり,例えば肺癌(1,490),脳腫瘍(806),頭頸部癌(726),前立腺癌(454),膵癌(393),アルツハイマー病(90),脳動脈瘤(35)[( )内はPuBMedでradiomics+疾患名(過去5年以内)で検索した結果の論文数,2024年8月30日現在]などに及ぶ。予測対象の例としては,生存リスク,再発リスク,悪性度,遺伝子変異予測,疾患の進行度予測である。レディオミクスは主に特徴量の計算と機械学習のステップからなる。現在のところ,レディオミクス特徴量には主に設計特徴量(engineered features)と自動特徴量(deep features)の2種類がある(図1)。設計特徴量は,特徴量の式[f(x)(x:入力画像)]やあらかじめ決めたアルゴリズムを用いて計算される2)3)。自動特徴量は,ディープラーニングのネットワークで決まる式[y=f(w,h,x),w:ネットワークの重み係数,h:ハイパーパラメータ]を用いて計算される4)。ディープラーニングでは自動的に特徴量を求めるため,ブラックボックス問題5)がある。ただし,設計特徴量も医学的に説明可能とは限らないし,機械学習と組み合わせたアルゴリズムの場合,ブラックボックスとなりうる。
Radiomics can be a black box because we have faced many “good” results, the reasons for which have not been explained for radiomics predictions. This review addresses the histogram, texture, and topology features among engineered features in radiomics, which are considered to have a relatively better explainability. The authors included features that they developed on their own, which they believed to be explainable. The authors introduced some studies on radiomics that, in their opinion, attempted to explain the reasons for the predictions. Finally, we discuss the current problems in radiomics and provide perspectives on radiomics.
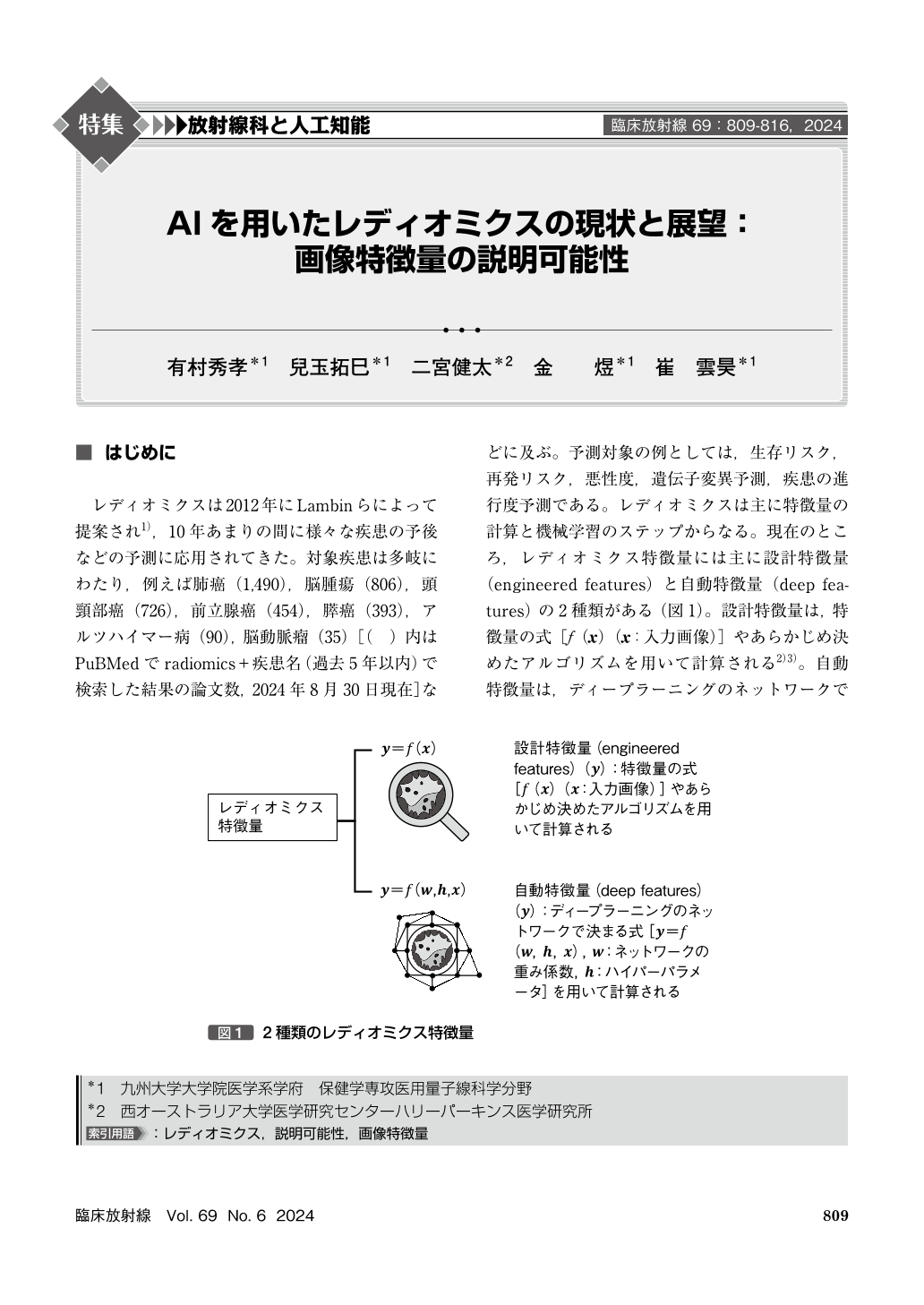
Copyright © 2024, KANEHARA SHUPPAN Co.LTD. All rights reserved.