Japanese
English
- 有料閲覧
- Abstract 文献概要
- 1ページ目 Look Inside
- 参考文献 Reference
がんは分子レベルで不均一な腫瘍である1)2)。例えば,肺癌ではゲノム不安定性(genomic instability),構造的染色体不安定性(structural chromosomal instability),腫瘍遺伝子変異量(tumor mutational burden),体細胞変異(somatic mutations),突然変異対立遺伝子の特異的不均衡(mutant allele specific imbalance),エピジェネティック不均一性(epigenetic heterogeneity)が腫瘍不均一の原因であるといわれている2)。また,腫瘍内部の遺伝子を含む分子レベルでの不均一さは予後との関連が指摘されている1-4)。遺伝子は生物の細胞の性質と形の作り方を決めているため5),腫瘍内部の分子レベルの不均一さは最終的に表現型としての医用画像上の不均一なピクセル値分布として現れる6)7)。不均一なピクセル値の分布を定量評価するための数式(ヒストグラム解析やテクスチャー解析など)から画像特徴量を求め,それらと予測対象(例:腫瘍の悪性度や予後)との関係を学習した機械学習を用いて,対象を予測する技術を研究する分野がレディオミクス(radiomics)である。機械学習(ディープラーニングを含む)を用いるので,レディオミクスも医療AI(medical artificial intelligence)の一つと考えられる。
The research topics, which have been addressed in radiomics, include long-term and short-term survival prediction, tumor grade prediction, genetic mutation detection, recurrence prediction, therapeutic outcome prediction and monitoring, treatment selection, and so on. AI models generally work on classification problems and regression problems that can deal with continuous values. For instance, the long-term and short-term survival prediction and gene mutation detection are two-group classification problems, while the tumor grade prediction is a regression problem. The target diseases treated in this special issue include brain, lung, breast, gastrointestinal, and gynecological tumors. This article outlines the procedures, evaluation methods, and necessary terminology for radiomics and radiogenomics analyses.
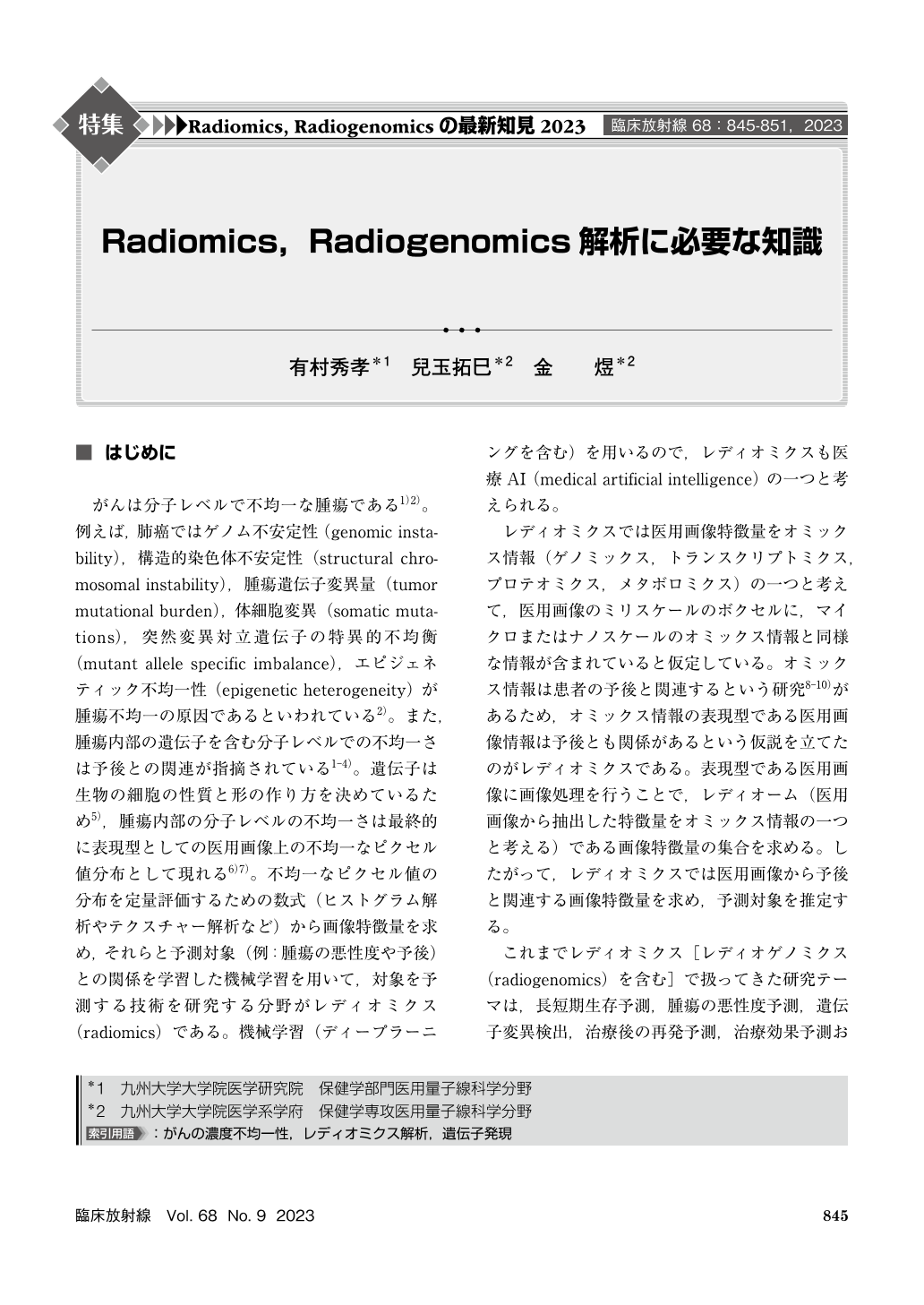
Copyright © 2023, KANEHARA SHUPPAN Co.LTD. All rights reserved.