Japanese
English
- 有料閲覧
- Abstract 文献概要
- 1ページ目 Look Inside
- 参考文献 Reference
模擬試験は受験生の学習到達状況を把握するうえで必要な手段であるが,模擬試験の点数のみでは十分とは言い難い.そこで,本学科で実施している模擬試験より,言語聴覚士国家試験の合格が危ぶまれる学生の抽出・対応を目的に,合否を予測する機械学習モデルの構築を行った.2010〜2018年度に本学科の養成課程を経て国家試験を受験した学生において,同一の模擬試験を受験した学生161名の解答データを対象とした.トレーニングデータとテストデータの4-fold交差検定を行い,モデルの予測結果と実際の合否結果においては有意な識別が行え(p<0.01),モデル精度は正解率0.74,適合率0.95,再現率0.71であった.また,模試の点数が最適カットオフ値より高いものの国家試験不合格となった受験者をすべて抽出することができた.本モデルは国家試験の合否予測に活用可能であり,到達状況が及ばない学生の発見・抽出により,国家試験対策を講じることができるツールとして使用可能と考える.
Practice tests are useful to grasp the status of students' learning achievement, but practice test scores alone are not sufficient measures. Using the data obtained from practice tests in our department, we constructed a machine-learning model that predicts the pass-fail status of the students who are at risk of not passing the national examination of speech-language-hearing therapists. The study used the data of 161 students trained in our department, who took the same practice tests and the national examination between 2010 and 2018. The 4-fold cross-validation of the training data and the test data was performed and significant discrimination between the model's prediction result and the actual pass-fail result was achieved (p<0.01), with a model accuracy of 0.74 for correctness, 0.95 for precision, and 0.71 for recall. In addition, all students who failed the national examination, even though their practice test scores were higher than the optimal cut-off value, were identified. This model can be used to predict the pass-fail result of the national examination and can be used as a tool to help at-risk students prepare for the national examination.
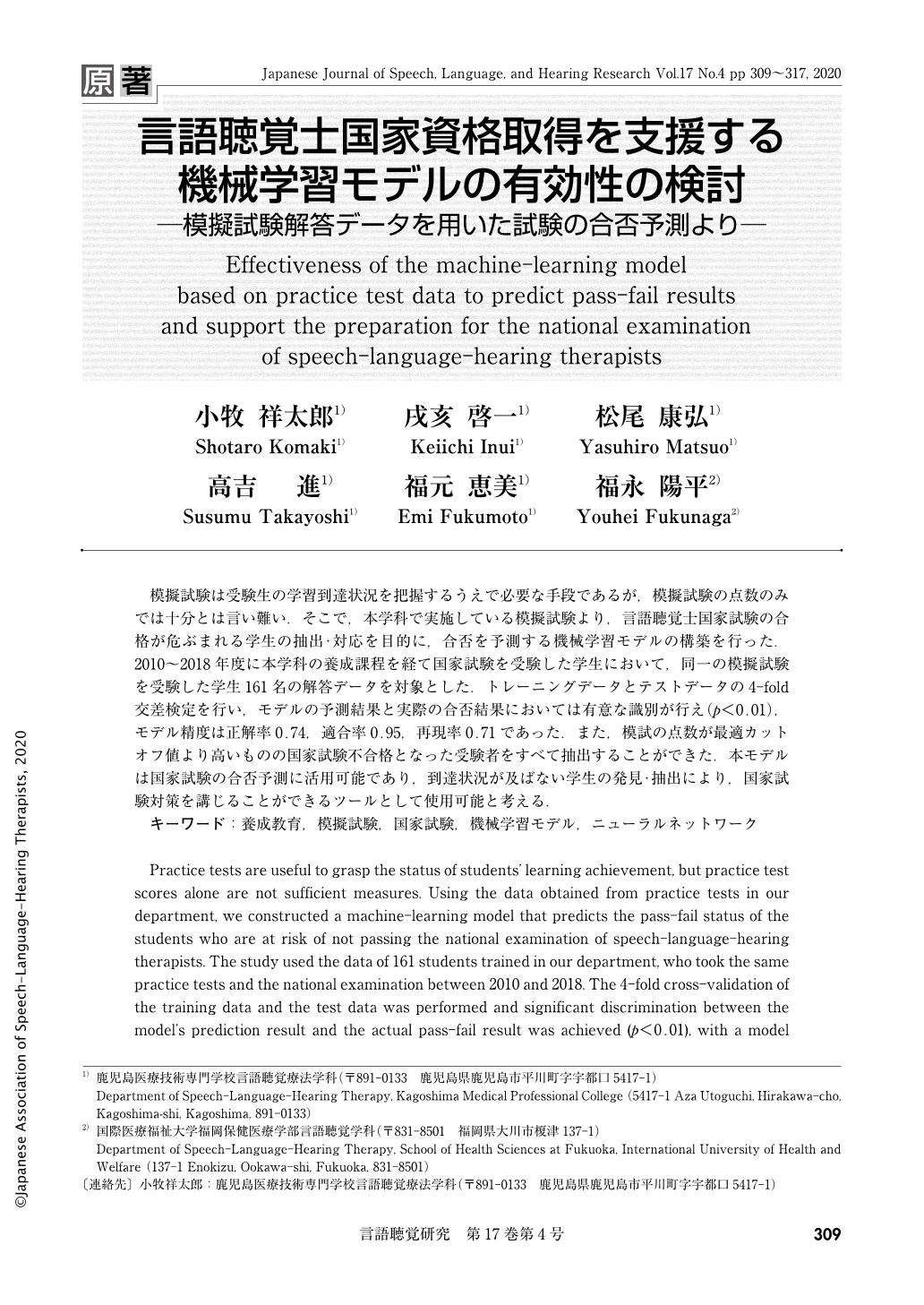
Copyright © 2020, Japanese Association of Speech-Language-Hearing Therapists. All rights reserved.