Japanese
English
- 有料閲覧
- Abstract 文献概要
- 1ページ目 Look Inside
- 参考文献 Reference
要旨●近年,消化管内視鏡領域におけるコンピュータによる補助診断(CAD)の応用は,畳み込みニューラルネットワーク(CNN)の発展に伴い急速に進歩している.胃癌に関連するCADの応用は,胃炎の鑑別診断,解剖学的部位の判別から始まり,胃癌の質的,量的診断と拾い上げ診断など多岐にわたって良好な成績が報告されつつある.一方,CNNを用いたCADには多量の学習用画像を必要とすることが多い.今回筆者らは少数の画像から切り出した微小領域のデータを拡張し,高効率な学習を可能とした胃癌の領域情報を提示するモデルを構築した.300画像から構築したCADにより,1年分の連続する胃癌患者137例462画像に対して画像ベースでの感度87.2%,症例ベースでの感度97.8%と良好な結果であった.高効率な学習を可能とするアプローチは,今後のCADの構築にも有用であると考えられた.
The use of CAD(computer-aided diagnosis)in gastrointestinal endoscopic imaging is rapidly progressing because of the development of CNN(the convolutional neural network). The application of CAD based on CNN has been developed from the recognition of anatomical locations and diagnoses for gastritis. Recently, researchers have reported favorable qualitative and quantitative results using CNN in the detection and diagnosis of gastric cancer. Whereas a large amount of data is required to construct a CNN model for CAD, we have developed a novel scheme involving automatic EGC detection with segmented localization. From a limited training dataset of only 300 images, we randomly cropped uncompressed small images and used data augmentation for transfer learning. Sensitivity rates for cases of 137 patients with cancer for one consecutive year and their related 462 images was 87.2%(image based)and 97.8%(case based). Our proposed scheme to use highly efficient learning can support CAD utilization in the early detection and future diagnoses of gastric cancer.
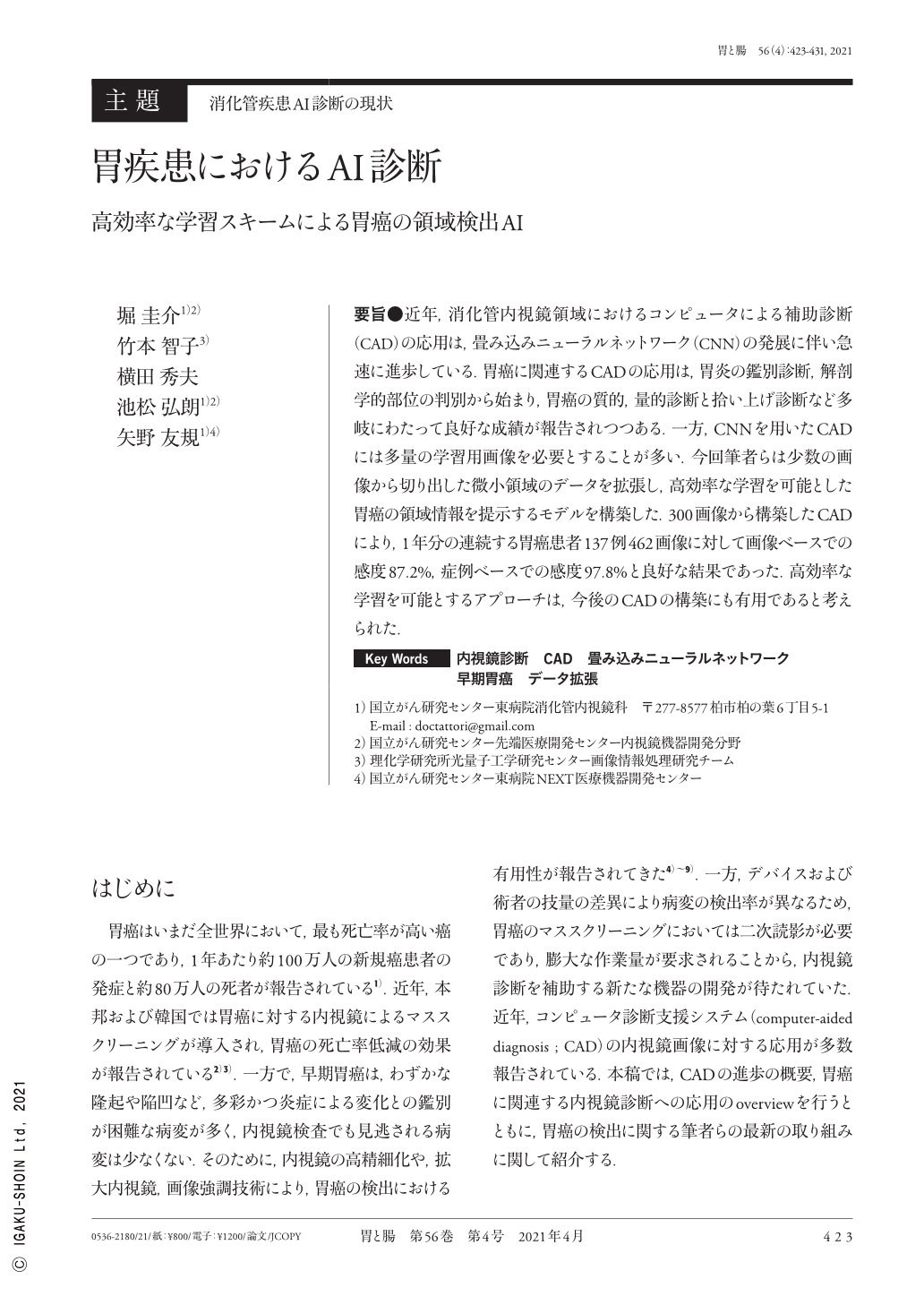
Copyright © 2021, Igaku-Shoin Ltd. All rights reserved.