Japanese
English
- 有料閲覧
- Abstract 文献概要
- 1ページ目 Look Inside
- 参考文献 Reference
要旨●胃疾患におけるAI診断の対象は,H. pylori感染診断,病変の拾い上げ,胃癌の質的診断・深達度診断など多岐にわたり,特にAIによる胃癌の拾い上げ診断,質的診断に関しては多くの研究が報告されている.また,胃拡大内視鏡を用いたAI画像診断支援システムも少なからず報告されており,今回は胃拡大内視鏡AI画像診断支援システムによる胃癌診断の現状について解説する.筆者らが開発した胃拡大内視鏡AI画像診断支援システムは,フルズーム浸水法を特徴としており,正診率98.7%,感度98%,特異度100%,陽性的中率100%,陰性的中率96.8%であり,既報の中で最も精度の高いシステムを開発することができた.しかし,リアルタイムに撮影される動画を用いた診断精度は不十分であり,今後さらなる改良が必要である.日常臨床での内視鏡医の診断と同様,通常観察(白色光)でのAI胃癌診断にも限界があることから,胃拡大内視鏡AI画像診断支援システムの上乗せ効果が予想されており,早期の実用化が期待される.
*本論文中、QRコードを読み込む,もしくはURLにアクセスいただくことで動画を再生できます(公開期限:2024年4月).
We constructed an AI(artificial intelligence)-assisted CNN(convolutional neural network)CAD(computer-aided diagnosis)system, based on ME-NBI(magnifying endoscopy with narrow-band imaging)images to enable the diagnosis of EGC(early gastric cancer)and evaluated the diagnostic accuracy of this system. The AI-assisted CNN-CAD system(ResNet50)was trained and validated on a dataset of 5,574 ME-NBI images(3,797 EGCs and 1,777 non-cancerous mucosa and lesions). For the evaluation of diagnostic accuracy, a separate test dataset of 2,300 ME-NBI images(1,430 EGCs and 870 non-cancerous mucosa and lesions)was assessed using this system. The overall accuracy, sensitivity, specificity, positive predictive value, and negative predictive value of the AI-assisted CNN-CAD system were 98.7%, 98%, 100%, 100%, and 96.8%, respectively. All the misdiagnosed images of EGCs were of low quality or of superficially depressed and intestinal-type intramucosal cancers that were difficult to distinguish from gastritis, even by experienced endoscopists. The AI-assisted CNN-CAD system for ME-NBI diagnosis of EGC had a considerable diagnostic ability. This system may possess substantial potential for clinical application in the future that could facilitate ME-NBI diagnosis of EGC in actual clinical practice.
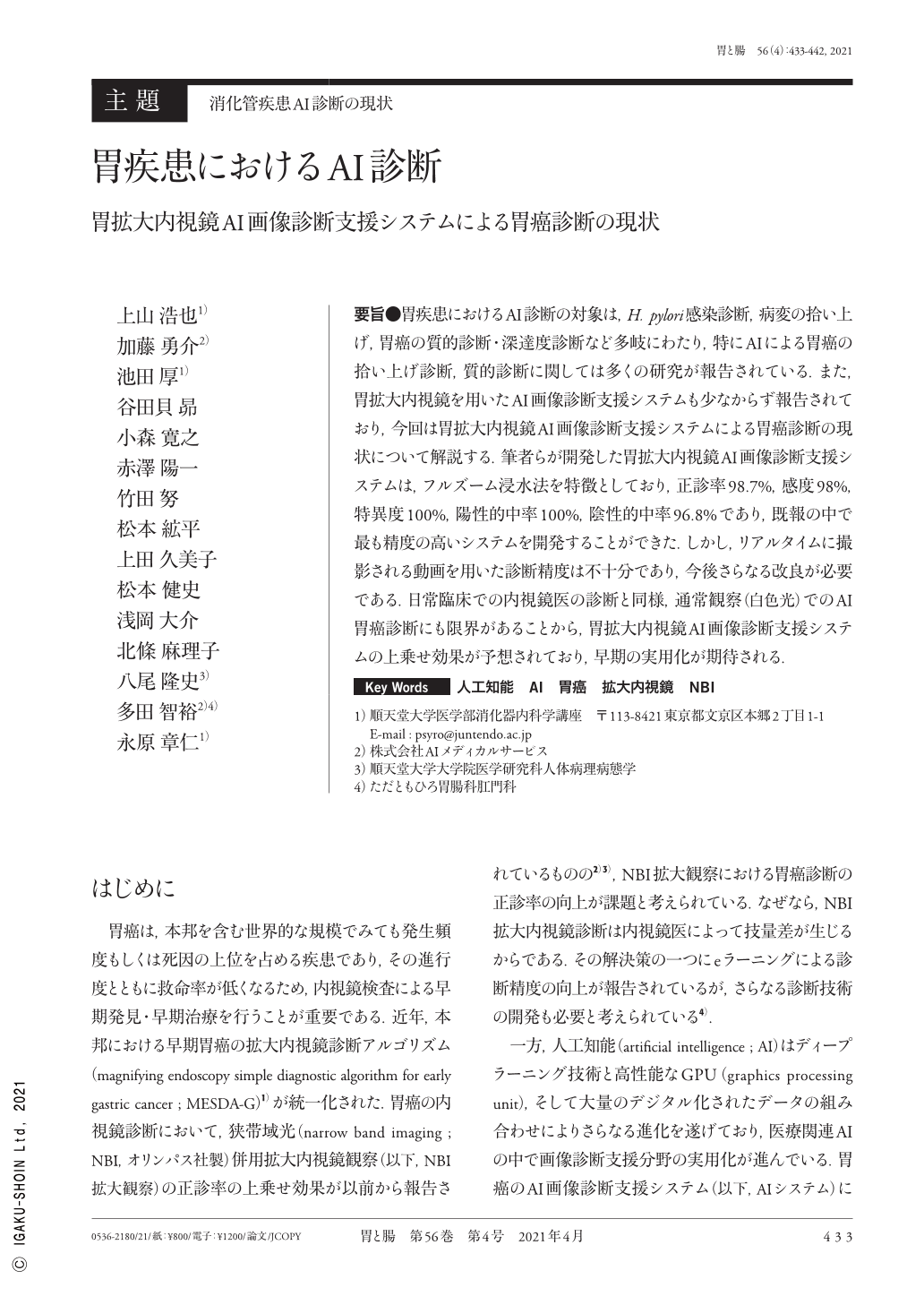
Copyright © 2021, Igaku-Shoin Ltd. All rights reserved.