Japanese
English
- 有料閲覧
- Abstract 文献概要
- 1ページ目 Look Inside
深層学習の「ゴッドファーザー」といわれているジェフリー・ヒントンが,社会に大きな変革をもたらした深層学習技術への貢献を認められノーベル物理学賞を受賞したことは記憶に新しい。特に近年,ChatGPT,Gemini,Claudeなどの生成AIサービスが一般に利用可能となり,我々の生活や仕事の幅広い分野で革新が進んでいる。これらのモデルは,文章作成や要約,翻訳といったタスクを迅速にこなすことができ,教育,ビジネス,エンターテインメントといった非医療分野においても広く応用されている。例えば,文章の自動生成を通じてブログ記事や報告書の執筆を支援し,長文を簡潔にまとめて要点を伝える要約作成が行われている。また,異なる言語間のコミュニケーションを容易にするための高精度な翻訳も提供されている。
This article discusses the potential and challenges of generative AI in healthcare, with a focus on radiology. Generative AI, including models like ChatGPT, offers tools to automate tasks such as summarization, translation, and report generation, reducing administrative burdens and improving efficiency. At Keio University Hospital, AI-driven tools are being developed to streamline clinical workflows, including discharge summaries, nursing summaries, and referral letters. These tools extract patient data from electronic records, providing automated outputs that can be customized by clinicians to fit departmental needs.
In radiology, AI is being used to identify incidental findings in imaging reports―unexpected abnormalities that require further investigation. However, ensuring that referring physicians do not overlook these findings has proven challenging. To address this, ChatGPT is being tested to flag critical findings and ensure they are properly documented. However, the precision of these systems requires continuous refinement, as prompts must be carefully adjusted to avoid both over-and under-detection of relevant conditions.
Despite its promise, generative AI faces challenges such as hallucination, where the AI generates inaccurate information. Keio University Hospital has encountered errors in AI-generated summaries, limiting their use to clinicians familiar with the patients to reduce risks. The hospital is also exploring Retrieval-Augmented Generation(RAG)to enhance the reliability of AI outputs by integrating external information.
Privacy concerns are another significant hurdle, as patient data handled through cloud-based AI services must comply with strict regulations. While cloud services offer access to the latest versions, on-premises solutions provide greater control and stability but require more resources.
In conclusion, generative AI holds promise for improving efficiency and decision-making in radiology. However, challenges related to accuracy, privacy, and system integration must be addressed to enable broader adoption in clinical practice.
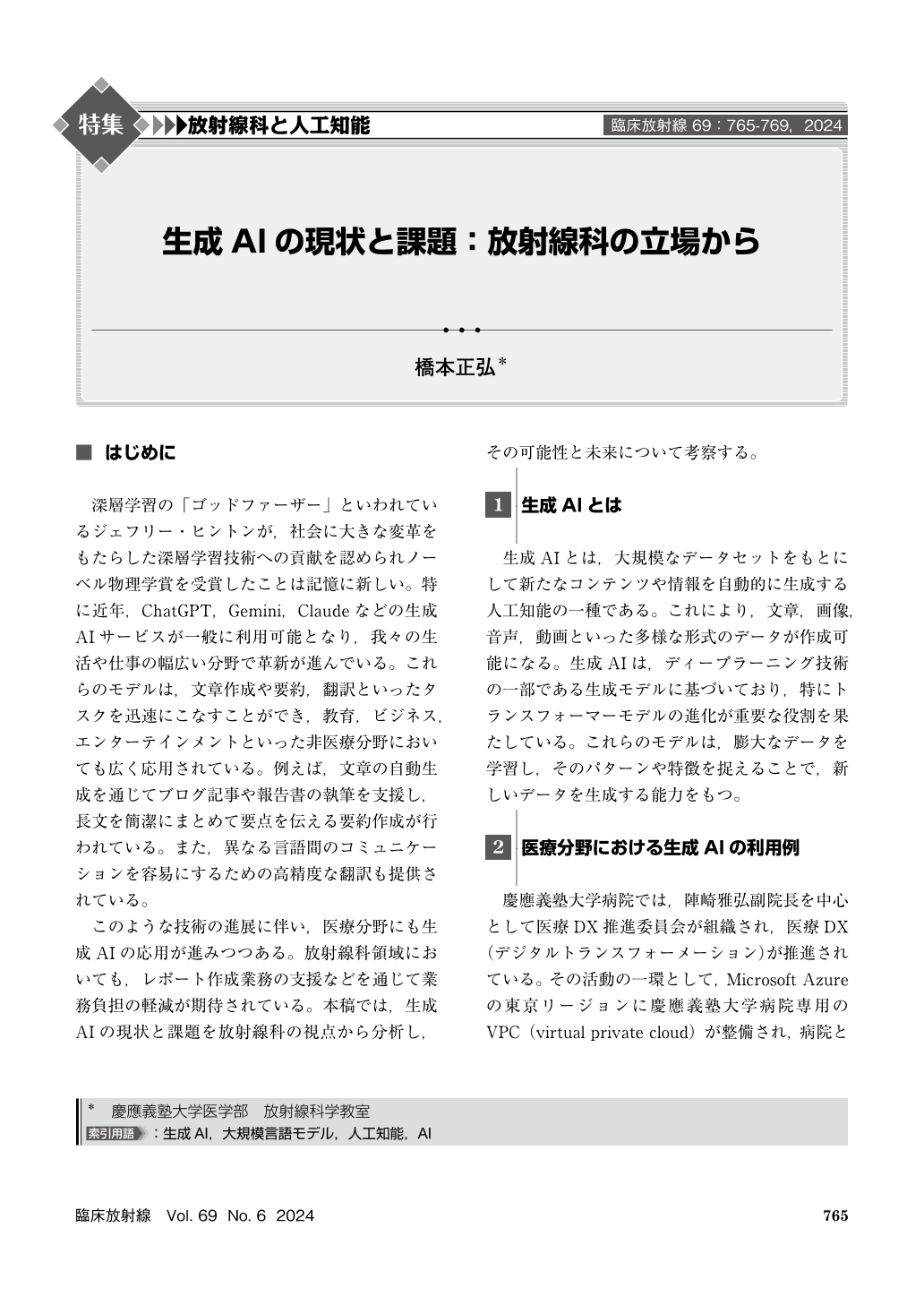
Copyright © 2024, KANEHARA SHUPPAN Co.LTD. All rights reserved.