Japanese
English
- 有料閲覧
- Abstract 文献概要
- 1ページ目 Look Inside
- 参考文献 Reference
機械学習によって複雑な計算・識別・予測を行うことが可能となったため,機械学習を用いた研究が急増している。機械学習は,教師なし学習(患者・疾患分類),教師あり学習(診断・予後予測),強化学習(判断支援)に分けることができる。教師なし学習は敗血症など従来単一概念であった疾患を細分化するために用いられ,集中治療において重要となると考えられる。一方,数多くの研究が教師あり学習を用いて予後予測を行っているが,現場への実装が大きな課題となっている。機械学習が今後さらに重要になることは間違いないが,臨床医にとって大事なことは,具体的な手法を学ぶことではなく,機械学習の得意・不得意を知り,今後の医療がどのように変わる可能性があるのか,そして患者のためになるのかを考えることである。
Machine learning can accurately perform complex calculations, classification, and predictions compared to conventional biostatistics, with techniques such as non-penalized logistic regression models. Machine learning is classified into unsupervised learning (classification), supervised learning (prediction), and reinforcement learning (decision-support). Unsupervised learning has been used to identify latent subgroups in those with a specific disease (e.g., sepsis), which has traditionally thought to be a homogeneous condition. Clinical research using unsupervised learning is expected to increase in the field of intensive care medicine. By contrast, while supervised learning is widely used to predict patient prognosis, implementation of developed algorithms becomes an important bottleneck in real clinical situations. Machine learning will be one of the most important techniques in clinical research. However, it is more important for clinicians to know the strengths and weaknesses of machine learning and to think about how machine learning can change the future of intensive care and how it can benefit patients, rather than learning specific techniques for machine learning.
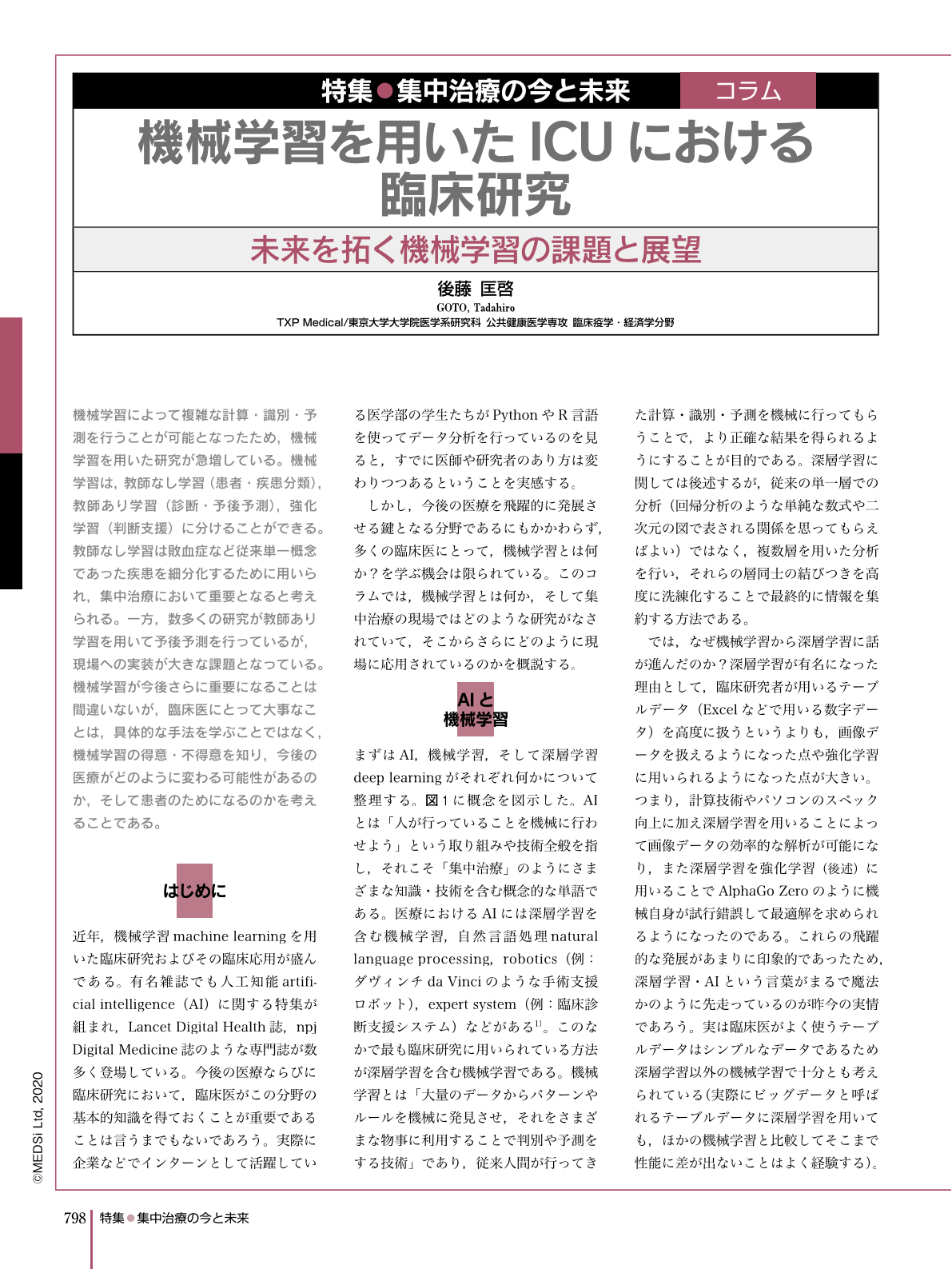
Copyright © 2020, MEDICAL SCIENCES INTERNATIONAL, LTD. All rights reserved.