Japanese
English
- 有料閲覧
- Abstract 文献概要
- 1ページ目 Look Inside
- 参考文献 Reference
半世紀前パーセプトロンを基にMarr-Albus-Ito小脳学習仮説が提案され,その検証過程で小脳学習と記憶の原因となるシナプス可塑性の長期抑圧(LTD)が発見された。小脳学習仮説は脳における深層学習の原型である液状態機械モデルへと発展し,運動のタイミングと効率の学習,運動記憶の形成や内部モデルに基づく認知機能まで拡張されつつある。パーセプトロンから発展した人工知能が今後小脳の神経科学に与える影響について論じる。
Abstract
Half a century ago, cerebellar learning models based on a simple perceptron were proposed independently by Marr and Albus. Soon, these models were combined with Ito's flocculus hypothesis that the cerebellar flocculus controls the vestibulo-ocular reflex through teacher signal-dependent learning, and consequently integrated into the so-called Marr-Albus-Ito cerebellar learning hypothesis. Ten years later, Ito found the synaptic plasticity of long-term depression at cerebellar Purkinje cell synapses, which underlies cerebellar learning. The liquid-state machine (LSM) model, which adds the random inhibitory recurrent neural network composed of granule cells --Golgi cells loop to a simple perceptron, explained the learning of timing in eyeblink conditioning, the learning of gains in ocular reflex, and the formation of short- and long-term motor memories in the cerebellum. The LSM model is now extended to the cerebellar internal model-based voluntary movement control and cognitive function. Artificial intelligence (AI) based on the neural network models originating from a simple perceptron, has now developed to deep learning. As the LSM model of the cerebellum is the counterpart of deep learning in the brain, the cerebellum is considered to be the origin of current AI. Finally, we discuss the impact of the evolution of AI on future clinical cerebellar neurology.
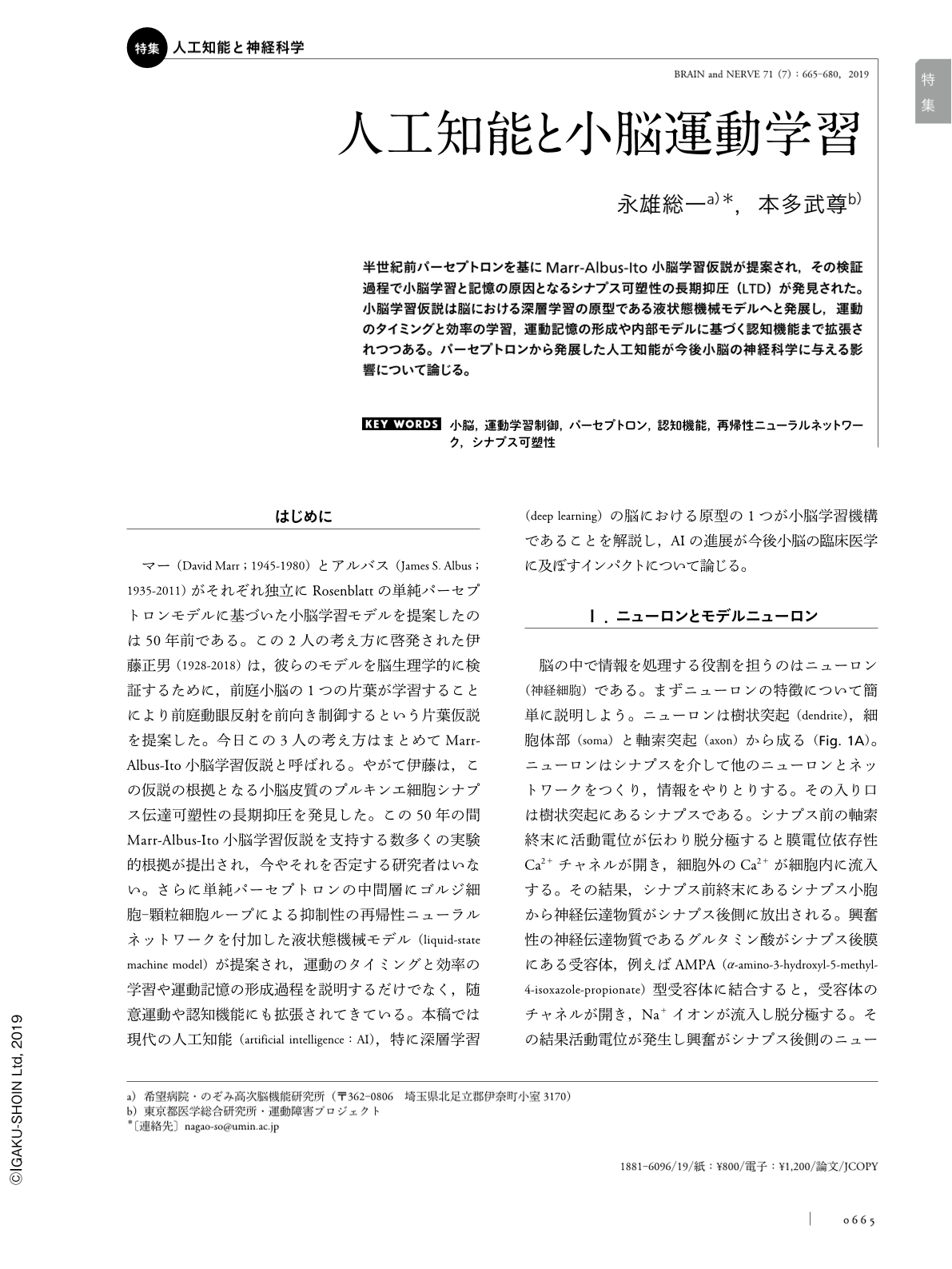
Copyright © 2019, Igaku-Shoin Ltd. All rights reserved.